As generative AI (genAI) continues to transform global business practices, ranging from optimizing workforce efficiency, reducing costs, and speeding up research and development, industry analysts are beginning to scrutinize its applications with a critical eye. This technology promises benefits for everyone from individual employees to executive leadership and shareholders, but could it be just as harmful as beneficial?
Due to genAI’s quick overtake, there’s virtually no national government oversight over how public or private companies wield this technology. Meanwhile, companies that lack established internal AI policies are scrambling to figure out how to leverage this transformative tech while ensuring it provides accurate information, enhances their specific workflows, and doesn’t produce misleading data that will require verification.
Ultimately, the output of generative AI models is only as good as the quality of the input.
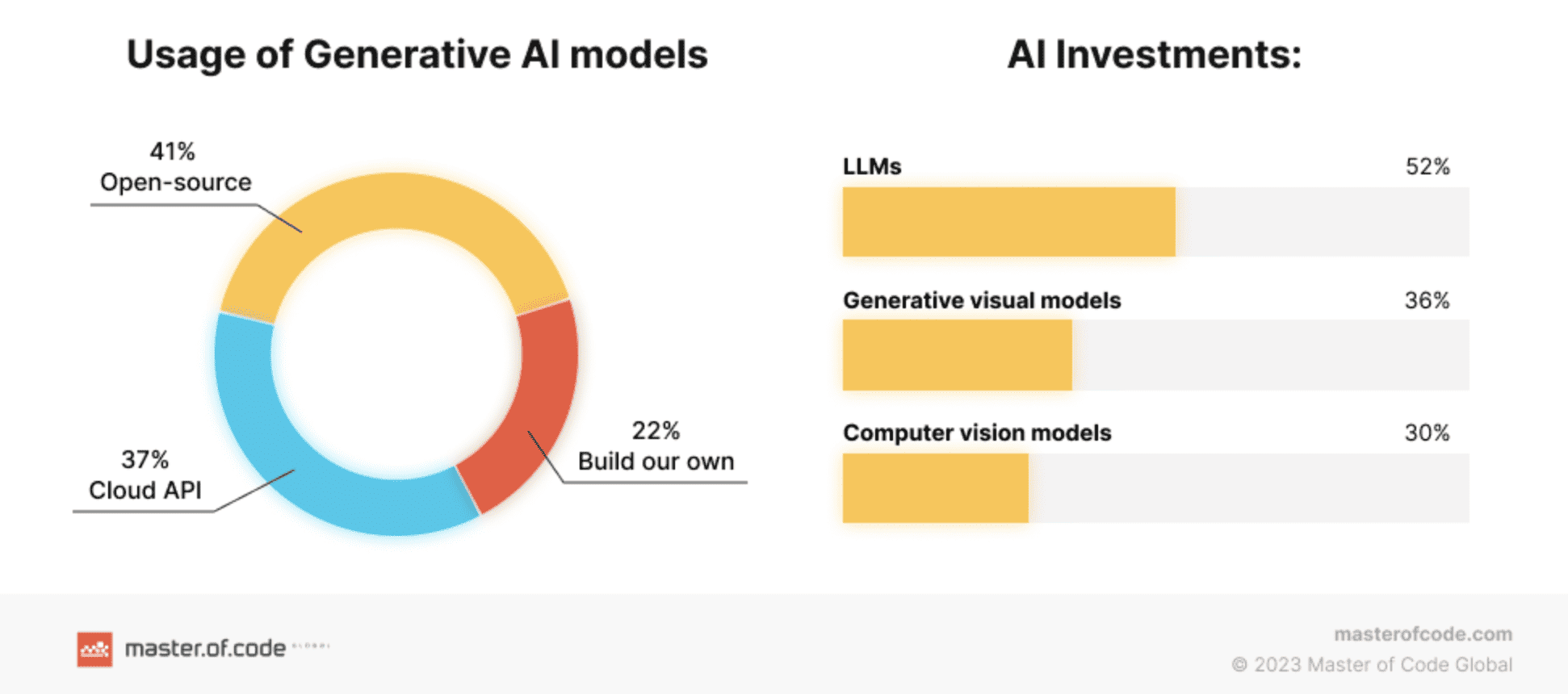
That’s why enterprise leaders who adopt genAI tools ensure that RAG, or retrieval-augmented generation, is integrated into them. RAG grounds a large language model (LLM)—a type of AI program that can recognize and generate text, among other capabilities—in authoritative content by asking it only to reason over information retrieved from a specified set of data, rather than reproduce knowledge from its training dataset.
But some C-level executives aren’t even sure what RAG is, let alone whether it’s a reliable piece of technology or how it can be used within a business context. Below, we answer the question left unaddressed in today’s genAI-centric world: can genAI and RAG be trusted to provide accurate and valuable information while protecting sensitive data?
Reliability in RAG
Originally developed by Meta, RAG merges retrieval-based models, which can access real-time and industry-specific data, with generative models that generate natural language responses. This hybrid method is especially advantageous in fields where current, domain-specific information is essential.
What RAG does is improve language models by integrating two essential parts: a retriever and a generator. The “retriever” of RAG locates pertinent information or documents from a vast dataset or corpus. It searches for data that can assist in responding to a query or aiding the generation process.
The “generator” or G of RAG is usually a language model (such as a variant of GPT) that produces text. It utilizes the information retrieved by the retriever component to generate more knowledgeable, precise, and contextually appropriate responses.
Typically, RAG grounds an LLM in authoritative content by asking it only to reason over information retrieved from a database rather than reproduce knowledge from its training dataset. This capability becomes essential to building trust and confidence in your genAI tools, as it sorts out misinformation from the accurate answers you need.
For example, ChatGPT, which is trained on the entire internet, maintains all of the benefits (i.e., access to all published human knowledge) and downsides (i.e., access to misleading, copyrighted, unsafe content) of the open internet. Within a business context, using ChatGPT introduces a level of risk that is unacceptable for work that informs critical investments and strategy decisions.
Current LLMs can effectively process thousands of words as input context for RAG, but nearly every real-life application must process many orders of magnitude more content than that. When it comes to AlphaSense, our database contains hundreds of billions of words, which makes the task of retrieving the right context to feed the LLM a critical step.
Open source—software whose source code is published and made available to the public, enabling anyone to copy, modify and redistribute the source—hasn’t caught up fully to closed frontier models in published performance benchmarks. However, it’s clearly leap-frogged closed models on the set of tradeoffs that any developer has to make when bringing a product into the real world.
The bottom line: open source software, or consumer-grade (trained on the internet) genAI tools may have larger datasets to train from, but this does not make them more intelligent or reliable—especially if RAG is not involved. For many tasks in your enterprise or investment context, your unique data assets and understanding of the problem offer a leg up on closed models.
RAG for Business-Specific GenAI
In the age of rapid genAI adoption, the reality of making misinformed business decisions based on inaccurate data will be commonplace amongst C-Suite executives who fail to not only acknowledge, but take proactive steps to rectify genAI’s faults. Accuracy is important for maximizing the value of your research and strategy-building—the more consistently and reliably you can access reliable, accurate intelligence from your tools, the more you can rely on them to take on the bulk of your work.
But, if your tools prove to consistently generate inaccurate answers, then they’ll never be useful enough to actualize that productivity and economic potential. On the other hand, specialization plays a critical role in one’s research. While one tool may work well for a generic use case (i.e., writing emails, summarizing meeting notes, answering surface-level questions about a company) it will often fall short in more specific or nuanced areas of your workflow.
For example, while a genAI tool can produce a reasonable or convincing answer, it’s ultimately worthless without the ability to verify its credibility and investigate the sources it references. That’s where consistent accuracy, specialized knowledge, and transparent workflows provide actualized value to organizations in a business context.
Collecting training data and regularly re-training a fixed model baseline enables systematic evaluations of model performance over time. With Smart Summaries, we provide our clients with citations to the exact snippets of text from where the platform’s summaries are sourced, combining high accuracy with instant and easy verification.
Ultimately, new technology adoption in enterprise requires meaningful investment in hardware and software frameworks that take time to plan, create, and implement. For genAI, obstacles include technological integration with existing systems, identification and implementation of security protocols, and obtaining and utilizing high-quality data. Once these obstacles are resolved, governance, intellectual property issues, and the potential for regulation remain headwinds.
RAG in AlphaSense’s Enterprise-Grade GenAI
Our vision for genAI at AlphaSense has always focused around a purpose-built approach, taking everything we’ve learned about AI for business over the last decade and applying it to this new phase of development.
We’re looking at some of the major challenges in the generative AI space right now—misleading or out-of-date information, unclear sourcing tactics, and hallucinations—and tailoring our approach to tackle those challenges head-on by building a product that is purpose-built for the use cases of our professional clients.
Today, we’ve gleaned insights into our LLM development, model choice, training data generation, retrieval-augmented generation and user experience design that have enabled the expansion of our company summaries, industry summaries, and our open chat through Generative Search.
The bottom line: the best genAI tools for enterprise professionals should be fine-tuned. In other words, a genAI tool should leverage a trained LLM on a more specific dataset to optimize its performance for the desired tasks or workflows.
In the case of AlphaSense, we have an extensive library of business and financial content at our fingertips to train our models—a library that sources from over 10,000+ premium data sources and boasts information from roughly 50 thousand public companies worldwide and over 1.4 million private companies. AlphaSense also aggregates equity research from 1,000+ leading brokers, plus all of Wall Street’s top analyst firms, including Goldman Sachs, J.P. Morgan, and Morgan Stanley.
But we didn’t just fine-tune AlphaSense’s LLM and RAG on verticalized content. We also trained these models on specific tasks that our customers have to do everyday: everything from earnings analysis, competitive landscaping, SWOT analysis, and so much more.
Additionally, we leverage our existing AI search capabilities—including Smart Synonyms ™—to ensure that the platform is pulling the most relevant passages to answer a given query. This creates the dataset that our LLM reasons over in order to best answer your question or summarize a predefined set of values . And potentially the most valuable parts about anything produced by genAI in our platform is that every answer or generated output links back to the exact source text, so you can be confident about the credibility of each insight..
Get Trust in Your GenAI with Enterprise Intelligence
In today’s highly competitive business landscape, marked by increased economic uncertainty and disruption across industries, an enterprise’s resources hold greater value than ever before. It plays a pivotal role in safeguarding competitive advantages and ensuring not just survival, but prosperity.
Ultimately, it’s not a matter of if you should have a market intelligence tool at your disposal, but instead, deciding on one you trust to keep you on the cutting edge of investment ideas, industry dynamics and competitor developments.
AlphaSense goes beyond the expectation of a typical market intelligence platform, providing first-in-class insights by integrating our trusted genAI technologies with internal IP that would otherwise be hard to find, synthesize, and share.
Start a conversation today to explore how AlphaSense’s enterprise solution can improve the search and discovery of insights across your internal content with the power of generative AI.
Don’t miss our State of Generative AI & Market Intelligence Report 2023.
Start your free trial of AlphaSense today.